Generative AI does certainly mark a huge step forward into the way machines interact with and create content. Gone are the days of single-task executions; now, AI can produce entirely original and new content – from texts and images to music and even full interactive media. The prospect of generative AI has piqued the interest not only among technologists but also among artists and scientists and businesses alike.
What is generative AI, anyway? How does it work? In this article, we dive deep into how generative AI works, its technological basis, its vast applications, and what the future might hold.
1. What Is Generative AI?: The Technology That Creates
It is a form of artificial intelligence where content is generated anew, which could be in the form of text, images, sound, or even data. It does not mimic, as traditional AI systems do based on explicit instructions, nor does it perform like some other AI systems, which are analytical tasks. The generative AI system does not replicate content from data in their established forms but instead creates new, original, and often startlingly lifelike outputs.
A generative AI model is, fundamentally, trained on massive datasets and learns to grasp the structure, patterns, and nuances of the input. Trained, the models can generate new content retaining qualities from the original data and including new and novel elements.
Generative AI can be divided into types based on the algorithms:
a. Generative Adversarial Networks (GANs)
Perhaps the most famous example of generative AI, GANs work by cleverly creating a game of mimic between two neural networks-the generator and the discriminator. The generator’s role is to create new data, such as an image or a segment of text, whereas the discriminator assesses this very same new data to determine whether it is real, that is to say part of the original training data, or fake, meaning it has been generated by the AI.
It goes on presenting a tug-of-war scenario between the generator and discriminator. This forces both the networks to do better as time passes by. Eventually, the generator performs so well that the discriminator fails to distinguish between AI-generated data and real data. GANs find a wide application in generating images, producing video generation, and even generating photorealistic deepfakes.
b. Variational Autoencoders (VAEs)
VAEs work somewhat differently than GANs. Here, rather than competing with each other, VAE compresses the data into a latent space-low dimensional representation-and then reconstructs it. This makes the model capable of producing new data points on the basis of learned representations, thereby making VAE particularly helpful in generating images or videos, depending upon specific attributes.
c. Transformer-Based Models
Transformer-based models have been ruling the text-generation landscape lately with OpenAI’s GPT-3. They process text as a sequence of texts, attempting to learn their contextual relationship. However, the result is a considerably more sophisticated model of language, developed to generate highly coherent contextual text.
With those generative models, AI systems break out from the chains of having simple tasks; it can create, innovate, and even surprise. To clarify everything, however, it is of course necessary to go into the tech behind it.
2. The Technology Behind Generative AI
Deep down within this generative AI lies a complex interaction of technologies that have their roots deep within deep learning and neural networks. Just like a human brain, these systems learn and output patterns, make predictions, and generate new content.
a. Neural Networks: The Kernel of Intelligence
This reduces the technology of generative AI basically down to neural networks: it’s the basic building blocks. They are hugely complex networks of interconnected artificial neurons that process and pass information in a hierarchical way. Each neuron takes in some little bit of input, applies some mathematical function-many people call this an activation function-and sends out its output to another layer.
The neural networks in a generative AI model do not just take in data; instead, they use what they learn to create new material. This ability to learn and recognize complex patterns from the data that trains them is where it improves and fosters success.
b. Deep Learning: Learning at Multiple Levels
Deep learning is one of the subfields of machine learning. It involves neural networks having multiple layers; therefore, they are also termed “deep.” Each layer in a deep learning network learns a different level of abstraction, from low-level features like edges in an image up to high-level features like objects or scenes.
For example, for the image generation model, the first layers would probably be able to be able to detect simple elements, such as lines or colors. And deeper layers would start to capture more complex patterns, that of shapes or objects. By the last layers of the architecture, the model is likely to generate new images completely similar to those it has been trained on.
c. Latent Space: The Creativity Engine
Latent space refers to a lower-dimensional space wherein the model encodes data, but it’s hard to describe the same actually. In some way, it is a compression of reality wherein the model captures only those features that define a given dataset. Through that space, the AI can play around with its learned features and create new content .
For instance, a model trained on images of dogs can determine what the underlying structure was to make a dog a dog-that is, ears, fur, or even shape. One can change the points in latent space and generate new dogs that exist not in the physical world but are still realistic.
d. Transformer Models: A New Frontier in Text Generation
Generative AI models work on language like GPT-3, which are built on transformers. Transformers use a notion called attention that allows the model to emphasize the relative weight of different words in a sentence. That’s essentially where the whole difference between comprehension of contextual meaning and relationships in words is involved and why these models are so strong at generating close to almost human text.
Transformers don’t simply predict the next word in sequence based on probability; they actually look at the whole context of a sentence or paragraph. This results in outputs that are coherent, grammatically correct, and often indistinguishable from human-written text.
3. Applications of Generative AI: Revolutionizing Creativity and Beyond
Generative AI is transforming various industries, providing new creative tools for content creation, design, music, and so much more. Here are some of the most groundbreaking applications of generative AI:
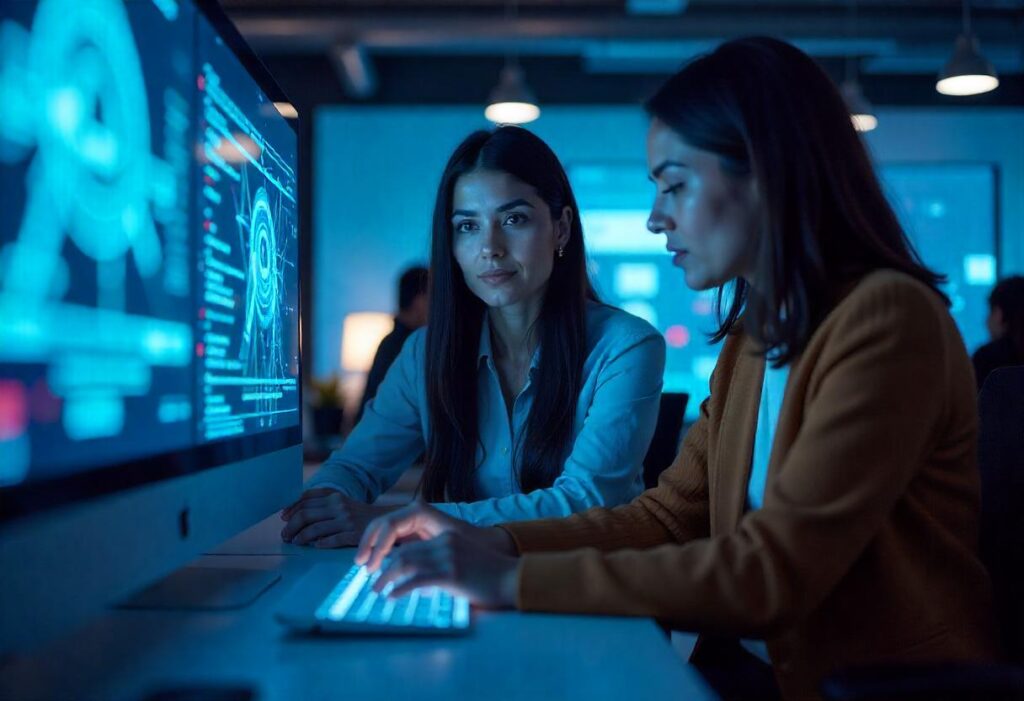
a. Content Generation
The most popular application of generative AI is text generation. GPT-3 and its peers demonstrate their ability to write articles, stories, essays, and even code. This unlocks an enormous scope of using AI in everyday working content for content creators, marketers, and businesses-to-write everything from blog posts to technical documentation.
For instance, a person can write a short prompt, then the AI will generate a long, coherent article. Tools such as Jasper AI and Copy.ai take advantage of this technology to automatically create content, hence lightening the burden on human writers with no compromise in quality.
b. Image Creation and Editing
Generative AI has done some brilliant work in the domains of generating and editing images. Such models, as that developed by Open AI known as DALL·E, have been able to generate completely new images based on text descriptions. For instance, a “a picture of a city in the future with floating cars” can culminate in a complete digital image.
This capability has great value in areas like fashion, advertising, and design. Designers can quickly prototype ideas, and marketers can make unique visualizations without the need for expensive photoshoots.
c. Sound and Music Composition
Generative AI also raises great noise in the music industry. An example is AIVA, an Artificial Intelligence Virtual Artist, an AI model able to compose original music of all varieties-from classical to jazz. These pieces can be used for films or video games, but can also be some background music in commercials.
Sometimes, composers can feed the model input and even use AI tools as collaborators to lead its creativity much like a conductor conducting an orchestra. This way, it not only accelerates the creative process but also generates new and unsought musical possibilities.
d. Video and Animation Generation
Generating video is another burgeoning field of generative AI. One can combine GANs with video data to create AI models that can generate video sequences. This can revolutionize industries such as gaming, where AI can create realistic characters and environments on the fly.
4. Case Studies: Generative AI in Action
Let’s take a few examples of real-world applications and look at how generative AI is changing the game across sectors.
a. GPT-3 for Content Creation
One of the most famous examples of generative AI is OpenAI’s GPT-3, a transformer model that has been trained on hundreds of gigabytes of text. GPT-3 can write text that very closely resembles human-written text, answer questions, compose essays, and even hold conversations. For instance, an organization like Copy.ai uses GPT-3 to automate marketing content, allowing businesses to create ad copy, blog posts, and product descriptions with minimal human intervention.
b. NVIDIA’s GauGAN for Image Creation
This NVIDIA GauGAN is an AI tool used to generate images. It enables users to create realistic images from simple sketches. It fills in all the details and builds up landscapes, portraits, and even abstracts. Now, artists and designers can rapidly prototype ideas, making visual concepts just less necessary than spending hours doing manual design work.
c. DeepMind’s AlphaFold for Scientific Discovery
DeepMind’s AlphaFold is, so far, the first true generative AI applied to scientific research. BetaFold predicts the three-dimensional structure of proteins based on AI, crucial to discovery of drugs and even understanding diseases. Already, this generative model has solved some of the most difficult challenges in biology, which could potentially accelerate medical progress by years.
5. The Future of Generative AI: A World of Infinite Possibilities
The future of generative AI is very promising, and as the technology unfolds at a fast pace, even more complex models are expected to pop out. These will be producing the highest level of content that would be able to stand beside what humans have created.
a. Human-AI Collaboration
Generative AI will increasingly become a collaborator instead of just a tool. The writer, musician, and artist will embrace their creativity with the intellect of AI by interlaying precision with machine intuition to create original works. It will unleash whole new genres of art, music, and literature.
b. Ethical and Societal Implications
More powerful generative AI automatically raises the ethics of such capabilities. Misinformation deepfakes can be proliferated; AI-generated content may replace humans as the creators of artistic works. Increasingly ubiquitous AI-generated media brings its own questions into the social arena involving authenticity, ownership, and regulation.
c. Personalized Content with AI
In the future, we’ll be able to use generative AI in personalizing content according to individual preferences. For instance, it can compose articles, music, or artwork based on a user’s interactions. This may be a source of hyper-personalized entertainment experiences where each movie, song, or book is specifically generated for each consumer.
Conclusion: The Generative AI Revolution
While being more than the next tech revolution, generative AI is a creative revolution, pushing forward deep learning, neural networks, and sophisticated algorithms so that AI today can now create completely new content in which human and machine creativity are interwoven. Generative AI is changing industries-from writing and design to music and scientific discovery-and pushing out frontiers of what machines can do.
All things being equal, the future possibilities afforded by generative AI seem to be without number. Whether it is to co-create with artists, to automate content production, or unlock scientific discoveries, generative AI holds the key to a world where not only can machines be instructed but also create.